Radiomics: A quantitative approach for high precision diagnostic imaging
Research Highlight | November 13, 2020
This article is an excerpt from the research magazine “Tackling Global Issues vol.2 New Era of Radiation Therapy to Fight Cancer.” Click here to see the table of contents.
Khin Khin Tha pursues the study of radiomics, a relatively new field which applies comprehensive and quantitative data analyses on MRI images to achieve more accurate diagnosis.
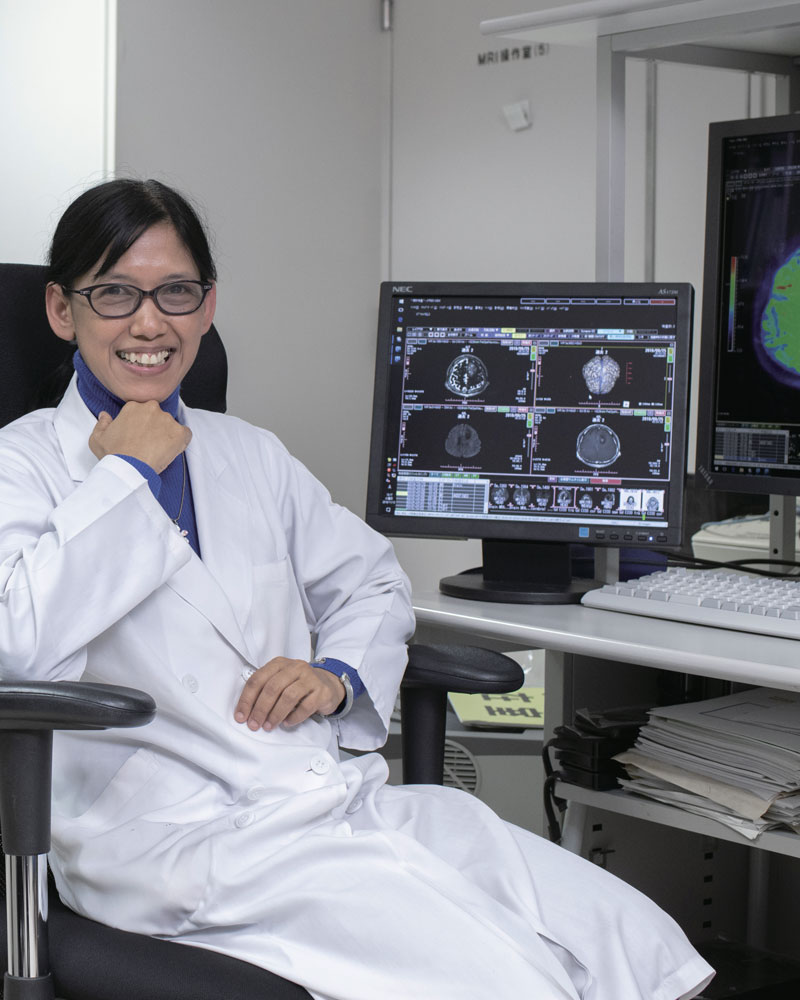
A Specialist of diagnostic radiology and neuroradiology, Dr. Khin Khin Tha is a Senior Assistant Professor at Hokkaido University Hospital. She received her MD (MBBS) from University of Medicine (2), Yangon, Myanmar
Since its debut in the late 1970s, magnetic resonance imaging (MRI) has helped doctors diagnose everything from sprained ankles to cancer in millions of patients. While computed tomography (CT) scans can provide images quickly and are highly suited for identifying bone fractures and calcification, and positron emission tomography (PET) scans are capable of visualizing metabolic processes within the body, the MRI scan’s non-invasive nature and lack of radiation exposure can make it the ideal choice for patients who require multiple scans. The MRI also provides finer anatomical details than both the CT scan and the PET scan, making it the superior choice in helping doctors diagnose patients with certain diseases.
However, for all the information an MRI can provide, there are still weak spots when it comes to the analytical appraisal of the scan. Radiologist Khin Khin Tha of Hokkaido University Hospital’s Department of Diagnostic and Interventional Radiology explains, “Until now, radiologists have tended to evaluate MRI results with our eyes instead of measurements. This is a fast and reliable method of analysis for radiologists working on the clinical side but is sometimes inadequate for research purposes when exact data is necessary, as a visual-only analysis can carry the risk of results being influenced by the radiologist’s bias.” After receiving her Ph. D in radiology at Hokkaido University in 2005, Tha has focused her research on applying quantitative analysis to MRI scans, as opposed to the commonly used and accepted visual analysis techniques. After meeting with a group of Stanford researchers, in 2015 she began working in what she describes as her “dream field”: the newly developed field of radiomics.
A new method for accurate diagnosis
Developed about 10 years ago, radiomics is a technique that combines image and comprehensive data processing. It allows radiologists to obtain large amounts of quantitative data from an MRI image that are impossible to gather through a purely visual inspection of an MRI scan. Radiologists upload the MRI results into a system that analyzes the images and cross-references them within a large database of other related tumors, providing comprehensive information on the tumor’s quantitative features, such as signal intensity characteristics, surface area, texture, and volume. For cancer patients suffering from glioblastoma, the most common and usually aggressive type of brain cancer found mainly in adults, MRI scans conducted utilizing radiomic techniques were able to help radiologists identify substantial differences in features within the same tumor that had visually appeared to be homogeneous on a standard scan. “Before radiomics, radiologists were satisfied with their visual assessment because they believed their own eyes,” Tha explains. “However, through radiomics, we are able to breakdown and comprehensively analyze different parts of a tumor without performing an invasive biopsy.”
Without using radiomic techniques, the only way to accurately assess certain features of a glioblastoma tumor was to extract a section of the tumor itself for analysis. With radiomics, doctors can fully characterize a tumor by obtaining data regarding the intensity, shape, and texture of the full tumor from just an MRI scan, providing vital information about the tumor’s aggressiveness and helping doctors create individualized treatment plans for the patient. This is especially important due to a phenomenon known as intra-tumor heterogeneity, in which diverse types of cancer cells behave differently within the same tumor, providing a severe roadblock to effective treatment methods. Due to this phenomenon, even a physical biopsy is sometimes not enough to fully understand the characteristics of a glioblastoma tumor, as the biopsied section may not have been representative of the tumor as a whole. Radiomics helps solve this issue by giving radiologists and doctors nearly all the information they need to assess the tumor, in best-case scenarios down to its genetic sub-type, and deliver an accurate prognosis and treatment regimen.
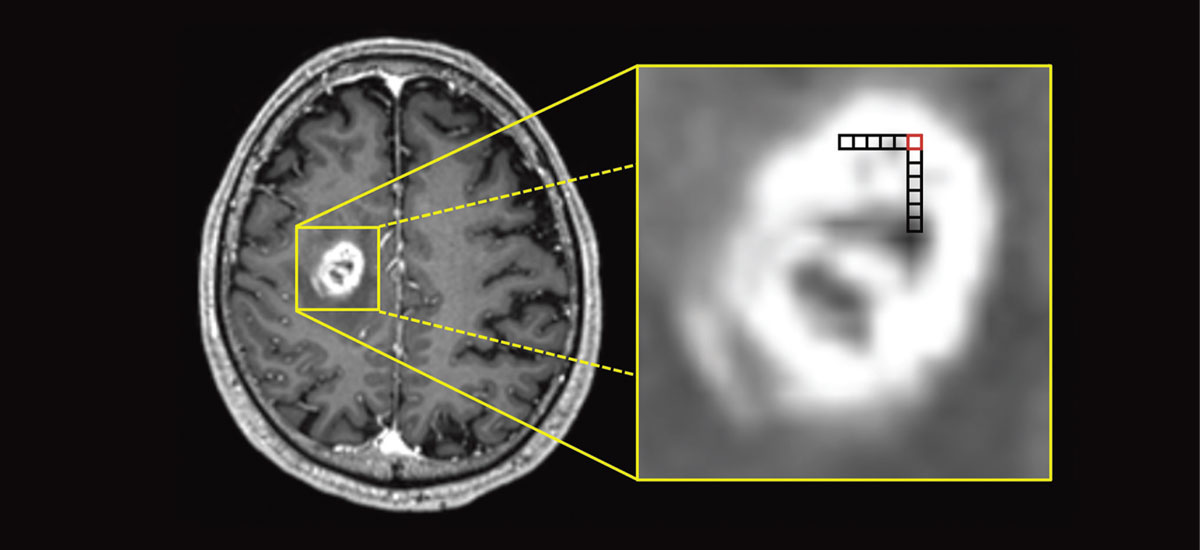
A standard MRI scan of a glioblastoma tumor (left). By applying radiomic analytics to the scan, the image can be broken into individual voxels (right), which can independently verify specific quantitative features of the tumor, helping doctors better understand its inner-complexities. This data can then be used to obtain a comprehensive view of the entire tumor, a feat which was previously impossible using only visual analysis methods.
A global team effort
In its current state, radiomics is a true team effort, requiring a collaboration of radiologists, computer scientists, clinicians, and biologists to derive the required results. Due to the necessity of this inter-disciplinary collaboration, it can sometimes be challenging to obtain the necessary expertise to advance research forward. Through the GI-CoRE program, Hokkaido University partnered with Stanford University, creating a mutually beneficial research environment where both universities are able to take advantage of shared knowledge and resources. Together, this collaboration has published three research papers on applying radiomics to glioblastoma patients, with the results indicating that survival prognosis was better when radiomic techniques were used in assessing a patient’s tumor compared to using only conventional prognostic measurements and techniques.
Still, despite the promising results that radiomic analysis offers, there are still a multitude of challenges that must be overcome before the technology can gain widespread use. While the ultimate goal is to be able to have the MRI system itself conduct the processes necessary to obtain a radiomics report, in its current state radiologists must undertake additional image processing steps in order to receive the fully comprehensive data, which can take time. However, according to Tha, the main obstacle researchers are facing now comes down to data. “A large amount of data is needed to carry out a radiomic report,” she says. “However, currently this data is not always available due to differences in collection and treatment methods and a lack of standardization, not only between methodologies but also between the MRI scanning equipment itself. For my research on glioblastoma, there were also issues in data collection due to the relative rarity of the disease and different intervention practices between hospitals, with some hospitals intervening with aggressive treatments earlier than others.”
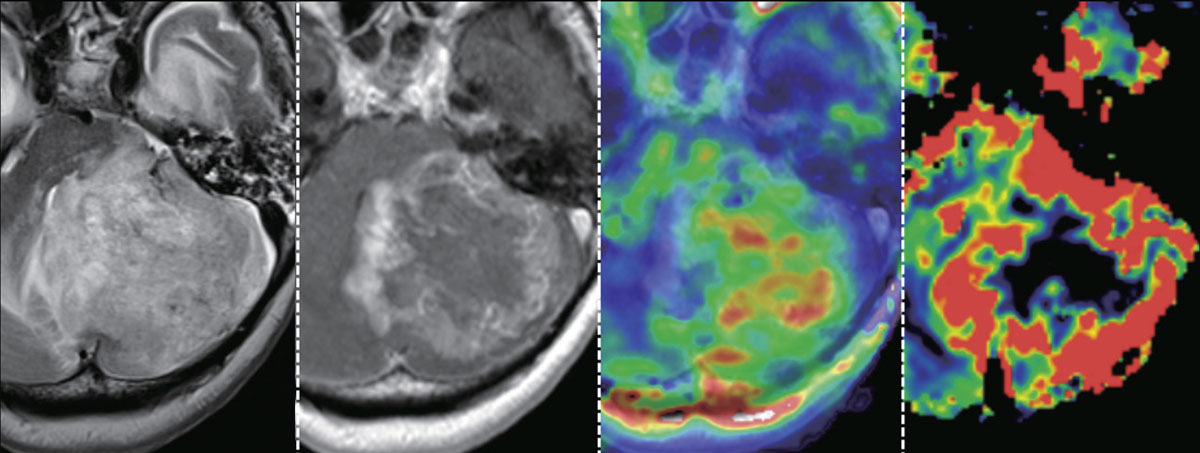
Different MRI datasets (from left to right, a T2-weighted image, a contrast-enhanced T1-weighted image, a mean diffusion kurtosis image, and a cerebral blood volume image) of a glioblastoma showing intratumor heterogeneity. The signal intensity on T2-weighted image, degree of contrast enhancement, mean diffusional kurtosis (which reflects tissue complexity at the microstructural level), and cerebral blood volume all vary greatly within the tumor.
Forging ahead in the field of radiomics
As more research is conducted, Tha hopes that one day radiomic assessment will be the standard in MRI-based cancer screenings, with machines completely capable of carrying out complex data analysis utilizing advanced AI technology under the guidance of a single operator. She also envisions a future where these techniques are applied to other diseases affecting not only the brain, but also different parts of the body. “We have already applied AI radiomic techniques to identify triple-negative breast cancers, which have the worst prognosis out of all breast cancer types. In addition, we are currently working on studies to use radiomics to help identify MRI features of Parkinson’s disease which would allow for a speedier diagnosis. Still, more work needs to be done, especially collaborative work, in order to gather the amount of data needed to implement radiomics on a wide-scale.”
Click here to see the table of contents.